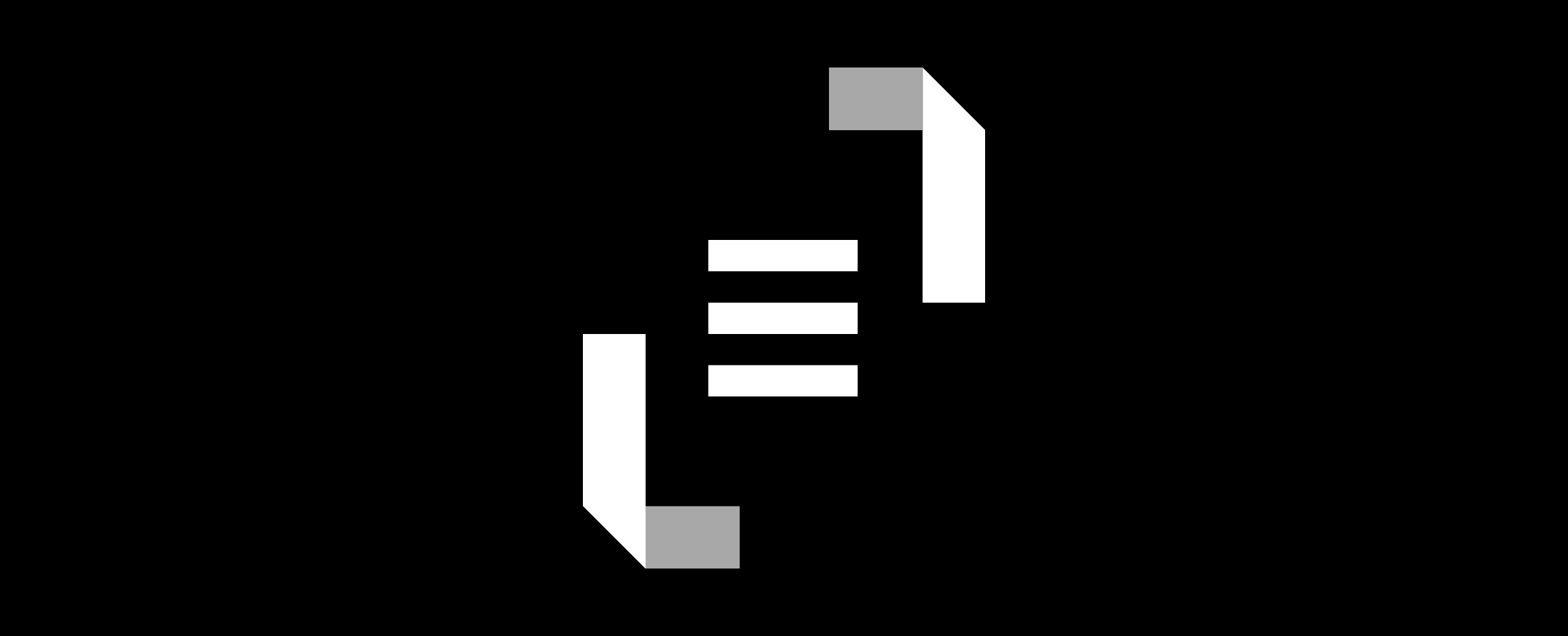
Matching Theory and Evidence on Covid-19 using a Stochastic Network SIR Model
Wednesday 11th November 2020
CINET:
2048
Pesaran, M. H. and Yang, C. F.
This paper develops an individual-based stochastic network SIR model for the empirical analysis of the Covid-19 pandemic. It derives moment conditions for the number of infected and active cases for single as well as multigroup epidemic models. These moment conditions are used to investigate identification and estimation of recovery and transmission rates. The paper then proposes simple moment-based rolling estimates and shows them to be fairly robust to the well-known under-reporting of infected cases. Empirical evidence on six European countries match the simulated outcomes, once the under-reporting of infected cases is addressed. It is estimated that the number of reported cases could be between 3 to 9 times lower than the actual numbers. Counterfactual analysis using calibrated models for Germany and UK show that early intervention in managing the infection is critical in bringing down the reproduction numbers below unity in a timely manner.
Keywords
Covid-19
multigroup SIR model
basic and effective reproduction numbers
rolling window estimates of the transmission rate
method of moments
calibration and counterfactual analysis
C13
C15
C31
D85
I18
J18
Themes
empirical