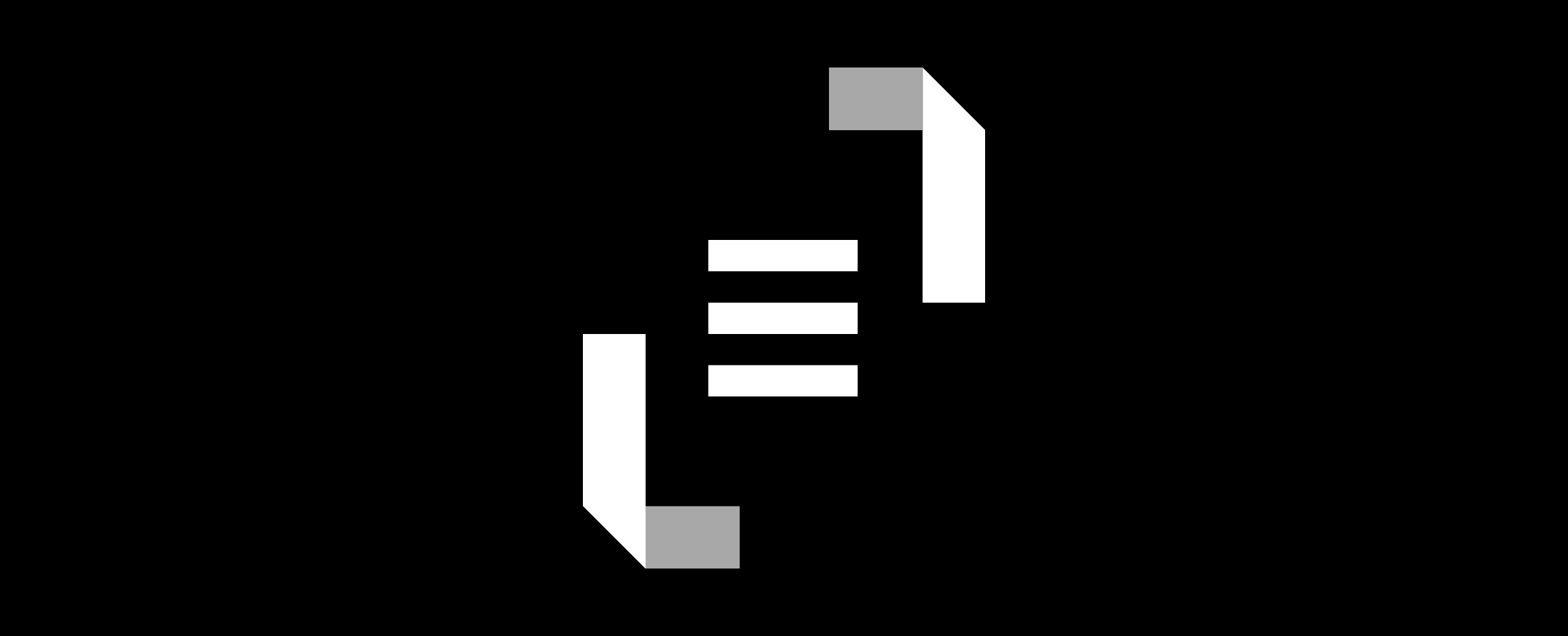
Semiparametric Single-index Predictive Regression
Thursday 12th September 2019
CINET:
1918
Zhou, W., Gao, J., Harris, D. and Kew, H.
This paper studies a semiparametric single-index predictive regression model with multiple nonstationary predictors that exhibit co-movement behaviour. Orthogonal series expansion is employed to approximate the unknown link function in the model and the estimator is derived from an optimization under constraint. The main finding includes two types of super-consistency rates for the estimators of the index parameter. The central limit theorem is established for a plug-in estimator of the unknown link function. In the empirical studies, we provide ample evidence in favor of nonlinear predictability of the stock return using four pairs of nonstationary predictors.
Keywords
Predictive regression
Single-index model
Hermite orthogonal estimation
Dual super-consistency rates
Co-moving predictors
C13
C14
C32
C51
Themes
empirical